In the era of rapid technological advancements, AI chatbots have emerged as a transformative force, reshaping the way we interact with machines and providing new opportunities for businesses. This article delves into the AI chatbot revolution, emphasizing the pivotal roles played by machine learning and large language models in this evolution.
The Rise of AI Chatbots
The journey from basic scripted responses to AI-driven chatbots has been nothing short of remarkable. AI chatbots have come a long way since their inception, transforming from simple, rule-based systems into intelligent conversational agents capable of understanding and generating human-like text.
AI chatbots first appeared in the form of rule-based systems with predefined responses. They could perform basic tasks, such as answering frequently asked questions or executing simple commands. These early chatbots lacked the ability to engage in meaningful, context-aware conversations.
However, as technology advanced, so did chatbots. Machine learning algorithms and natural language processing (NLP) techniques became integral to their development. These advancements allowed chatbots to analyze user inputs, interpret context, and generate more nuanced and contextually relevant responses.
Today’s AI chatbots can handle a wide range of tasks, from providing customer support to assisting with complex decision-making processes. They have become indispensable tools for businesses looking to enhance user experiences, streamline operations, and improve customer satisfaction.
The Role of Machine Learning in Chatbot Development
Machine learning is the driving force behind the sophistication of modern chatbots. It equips them with the ability to learn, adapt, and improve their interactions with users over time. But how does this work, and what types of machine learning techniques are involved?
Machine learning empowers chatbots by enabling them to:
1. Learn from Data
Chatbots are trained on vast datasets containing text and conversation samples. These datasets expose chatbots to diverse language patterns, helping them understand different ways users may phrase their questions or requests. The more data chatbots are exposed to, the better they become at understanding and responding to user inputs.
2. Adapt to User Input
Machine learning algorithms enable chatbots to adapt to the preferences and conversational style of individual users. As users interact with chatbots, the algorithms continuously analyze and learn from these interactions, allowing chatbots to tailor their responses for a more personalized experience.
3. Improve Over Time
One of the remarkable aspects of machine learning-driven chatbots is their capacity for continuous improvement. As they gather more data and receive feedback, they can refine their language understanding and conversational abilities. This ongoing learning process ensures that chatbots become more effective and accurate in their responses.
Large Language Models as a Game-Changer in Chatbot Technology
Large language models, such as GPT-3 (Generative Pre-trained Transformer 3), have marked a significant turning point in the chatbot landscape. Their ability to generate coherent and contextually relevant text has opened up exciting possibilities for chatbot applications.
These large language models are pre-trained on massive datasets containing text from the internet. This pre-training equips them with a deep understanding of language, grammar, context, and even some world knowledge. When integrated into chatbots, they can generate human-like responses, making conversations with chatbots feel more natural and engaging.
GPT-3, for example, has 175 billion parameters, making it one of the largest and most powerful language models to date. This immense size enables it to handle a wide array of tasks, from answering questions and translating languages to generating creative content like poetry and art.
In the next sections of this article, we’ll explore further the impact of large language models on chatbot development and how they are revolutionizing the AI chatbot landscape. Stay tuned as we delve deeper into the exciting world of AI chatbots.
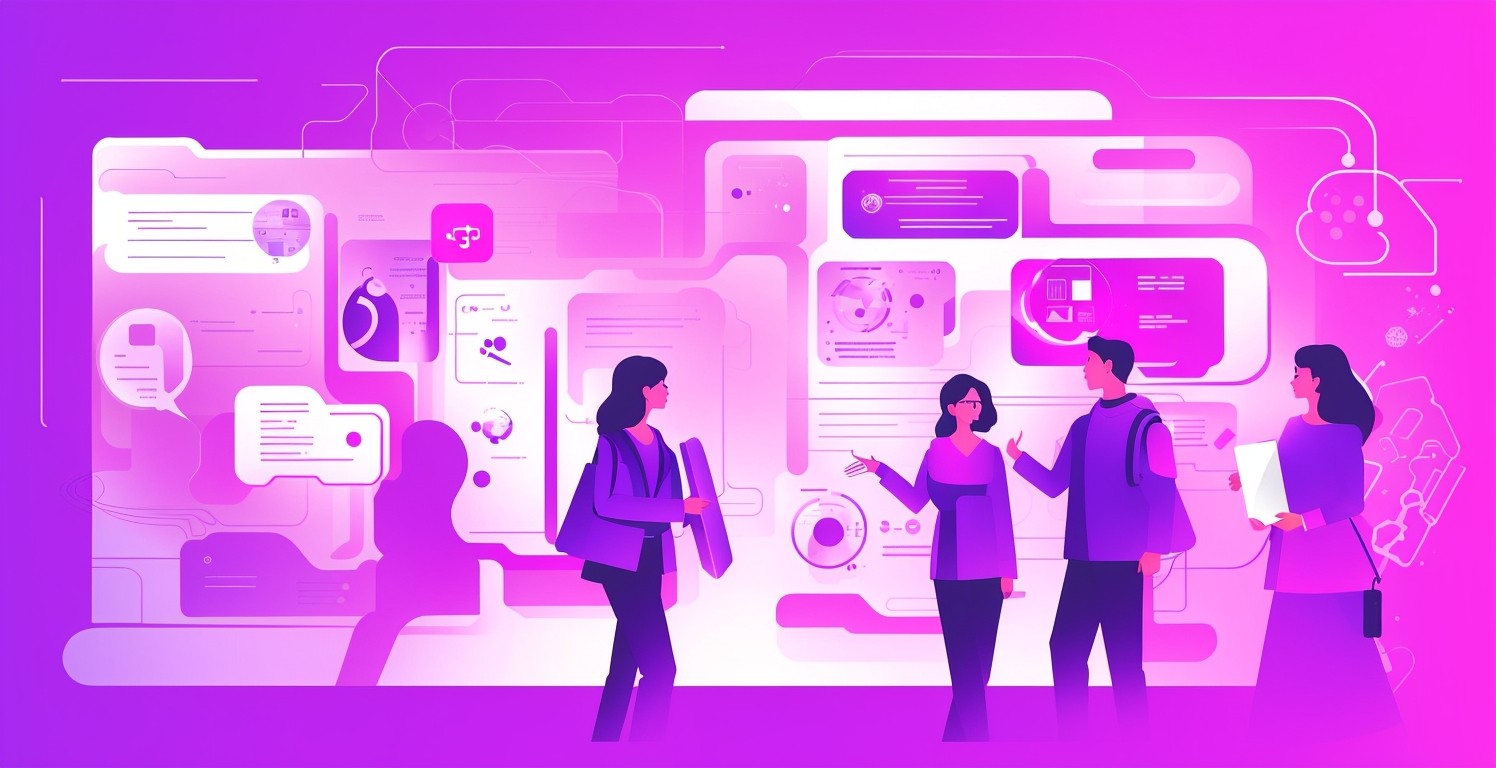
Understanding AI Chatbots: From Concept to Application
What Are AI Chatbots?
Artificial Intelligence (AI) chatbots, often simply referred to as chatbots, are computer programs designed to interact with humans through text or speech interfaces, simulating human-like conversations. These remarkable digital entities are powered by a combination of artificial intelligence and natural language processing (NLP) technologies. To truly grasp the significance of AI chatbots in today’s technological landscape, we must first understand their core components and capabilities.
The Inner Workings of AI Chatbots
At the heart of AI chatbots is artificial intelligence, which enables them to process, analyze, and generate human-like responses in real-time. NLP, a subset of AI, equips these chatbots with the ability to understand and generate human language, making them proficient in engaging in meaningful conversations.
The core functionalities of AI chatbots encompass:
- User Interaction: AI chatbots serve as intermediaries between users and computer systems. They can receive user input in the form of text or speech and respond accordingly, creating a dynamic, human-like conversation.
- Understanding Language: Through NLP, chatbots can decipher the nuances of human language. They can identify context, understand slang, and even recognize sentiment, allowing for more natural and context-aware interactions.
- Generating Responses: These chatbots are not limited to predefined responses. They can generate responses on the fly, drawing from vast datasets and their understanding of language. This ability makes their conversations feel more authentic and tailored to the user.
- Learning and Adaptation: AI chatbots are designed to learn from their interactions. They can adapt their responses based on user behavior and feedback, constantly improving their performance over time.
- Applications: The versatility of AI chatbots enables their deployment across various domains, including customer support, healthcare, finance, education, and more. They can handle tasks ranging from answering questions and providing recommendations to automating processes and assisting with complex problem-solving.
The Evolution of Chatbots
To fully appreciate the capabilities of modern AI chatbots, it’s essential to trace their evolutionary journey. Chatbots have come a long way from their rudimentary beginnings, and understanding this evolution sheds light on the immense progress achieved in the field of conversational AI.
The Early Years: Eliza and Rule-Based Systems
The history of chatbots can be traced back to the 1960s when the famous “Eliza” chatbot was created. Eliza, developed by Joseph Weizenbaum, was a simple rule-based program designed to mimic the behavior of a Rogerian psychotherapist. While limited in its conversational abilities, Eliza marked the inception of human-computer interaction through text-based conversations.
In the subsequent decades, chatbots remained primarily rule-based, relying on predefined scripts and pattern matching to generate responses. They were often used for specific tasks, such as providing technical support or acting as virtual assistants for scheduling appointments.
The Rise of Machine Learning: Smarter Conversational Agents
The turning point in chatbot development came with the integration of machine learning techniques. These techniques allowed chatbots to move beyond scripted responses and begin to understand user input more comprehensively. Chatbots started to learn from vast datasets of human interactions, improving their language comprehension and the quality of their responses.
This transition brought about more intelligent virtual assistants like Apple’s Siri, Microsoft’s Cortana, and Amazon’s Alexa, which could perform a wider range of tasks and provide more contextually relevant answers.
Modern AI Chatbots: The Age of Large Language Models
Today, AI chatbots have reached unprecedented levels of sophistication, thanks in large part to the emergence of large language models. These models, like GPT-3 (Generative Pre-trained Transformer 3), have transformed the chatbot landscape.
Large language models are pre-trained on massive datasets containing text from the internet, which equips them with a deep understanding of language and context. When integrated into chatbots, these models enable them to generate human-like responses with coherence and relevance, making interactions with chatbots feel remarkably natural.
Applications of Chatbots in Various Industries
AI chatbots are not confined to a single industry or use case. Their adaptability and versatility have led to widespread adoption across diverse sectors, revolutionizing how businesses and organizations interact with their audiences. Let’s explore some notable examples of chatbot deployments in various industries:
1. Customer Service and Support
In the realm of customer service, chatbots have become invaluable assets. They can handle routine inquiries, provide assistance with product selection, offer troubleshooting guidance, and even initiate returns or refunds. This automation of customer support tasks leads to quicker response times and enhanced customer satisfaction.
2. Healthcare
The healthcare industry has witnessed the integration of chatbots to streamline processes. Healthcare chatbots can schedule appointments, answer general medical questions, and offer medication reminders. Some advanced healthcare chatbots can even assess symptoms and provide initial triage recommendations.
3. Finance
Financial institutions deploy chatbots to provide customers with real-time information about their accounts, help with financial planning, and assist in processing transactions. Chatbots can also monitor financial markets and deliver personalized investment insights.
4. Education
In the field of education, chatbots offer tutoring, answer student queries, and provide educational content. They can adapt to individual learning styles, making education more accessible and tailored to the needs of students.
5. E-commerce
E-commerce chatbots assist users in finding products, making purchase decisions, and tracking orders. They can also recommend products based on user preferences and past interactions, enhancing the overall shopping experience.
6. Travel and Hospitality
In the travel industry, chatbots aid travelers by providing information about flights, accommodations, and local attractions. They can assist with booking reservations, offer travel tips, and handle itinerary changes.
These examples represent just a fraction of the industries where AI chatbots have made a significant impact. Their ability to automate tasks, provide timely information, and offer personalized assistance has led to increased efficiency and improved user experiences in numerous domains.
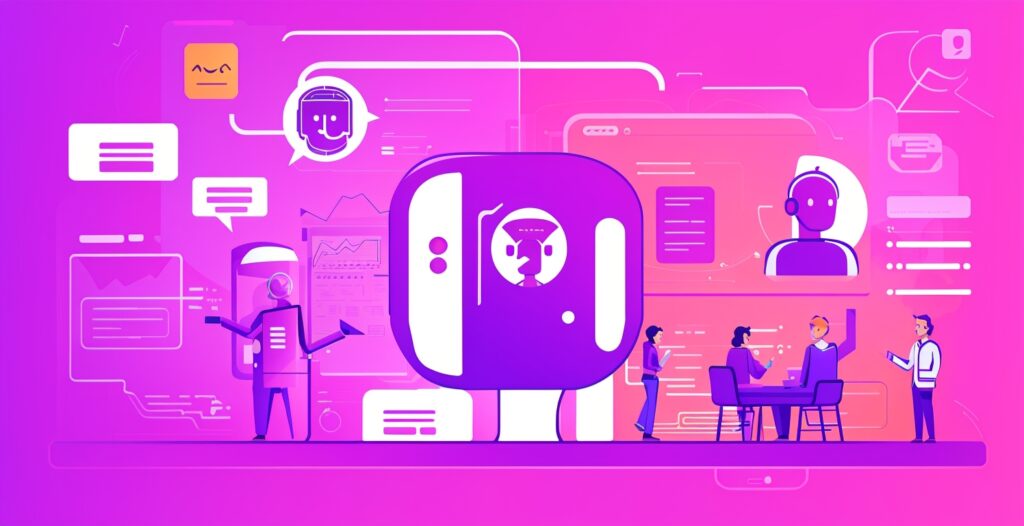
The Role of Machine Learning in Empowering AI Chatbots
As we continue our exploration of the AI chatbot revolution, it’s imperative to shine a spotlight on the pivotal role played by machine learning in enhancing chatbots’ capabilities. Machine learning is the driving force behind chatbot evolution, enabling them to learn, adapt, and provide increasingly personalized responses to users. In this section, we’ll delve into the mechanics of how machine learning empowers chatbots, the fundamental techniques involved, and its applications in chatbot development.
How Machine Learning Enables Chatbots
At its core, machine learning equips chatbots with the ability to improve their performance through data-driven learning. Unlike traditional rule-based chatbots, which rely on predefined scripts and patterns, machine learning-driven chatbots can:
1. Learn from Data
Machine learning empowers chatbots to learn from vast datasets containing user interactions, conversations, and contextual information. This data-driven learning process allows chatbots to recognize language patterns, understand user intent, and adapt their responses over time.
2. Adapt to User Input
One of the key strengths of machine learning-enabled chatbots is their adaptability. They can adjust their responses based on user behavior, preferences, and the context of the conversation. This adaptability results in more personalized and engaging interactions with users.
3. Improve Over Time
Machine learning-driven chatbots continuously refine their performance. As they accumulate more data and user interactions, they become more proficient at understanding user queries and providing relevant responses. This iterative improvement ensures that chatbots evolve and become increasingly intelligent.
4. Handle Ambiguity and Variability
Human language is inherently complex, filled with ambiguity, variability, and nuances. Machine learning equips chatbots with the capacity to navigate these intricacies. They can understand synonyms, handle misspellings, and recognize context, allowing for more natural and effective conversations.
Supervised Learning for Chatbot Training
Supervised learning is a fundamental machine learning technique used to train chatbots. In this approach, chatbot developers provide the model with labeled datasets, which consist of pairs of input (user queries or statements) and corresponding output (desired responses). The chatbot’s goal is to learn the mapping between inputs and outputs, enabling it to recognize and generate appropriate responses.
The Supervised Learning Process for Chatbots
- Data Collection: Developers gather a diverse dataset of user interactions, which includes a wide range of user queries and corresponding correct responses. This dataset forms the foundation for training the chatbot.
- Data Preprocessing: Data preprocessing involves cleaning and formatting the dataset to ensure consistency. This step may include removing duplicates, handling special characters, and tokenizing text (splitting it into meaningful units).
- Feature Extraction: Features, or characteristics, are extracted from the text to help the model understand the data better. This can involve techniques like word embedding, which represents words as numerical vectors.
- Model Training: The chatbot model, often a neural network architecture, is trained on the preprocessed dataset. During training, the model learns to predict the correct responses based on user inputs.
- Evaluation and Fine-Tuning: After training, the model’s performance is evaluated using a separate validation dataset. Fine-tuning adjustments are made to improve accuracy and responsiveness.
- Deployment: Once the chatbot achieves satisfactory performance, it is deployed for real-world interactions, where it can engage with users and provide assistance.
Supervised learning enables chatbots to handle specific tasks effectively, such as answering frequently asked questions or providing information about products and services.
Reinforcement Learning for Chatbot Optimization
Reinforcement learning takes chatbots to the next level by allowing them to refine their behavior through trial and error. This learning paradigm is inspired by behavioral psychology, where an agent (the chatbot) interacts with an environment (user interactions) to maximize a cumulative reward (providing valuable responses).
The Reinforcement Learning Process for Chatbots
- Agent-Environment Interaction: The chatbot interacts with users and receives feedback based on the quality of its responses. This feedback serves as a reward signal, indicating whether the chatbot’s actions were favorable or not.
- Policy and Action Selection: The chatbot employs a policy, a strategy for selecting actions (responses), to maximize the expected cumulative reward. It chooses actions that are likely to result in positive feedback.
- Learning from Feedback: Through iterative interactions, the chatbot learns which actions lead to better rewards and refines its policy accordingly. This process allows the chatbot to improve its conversational abilities and decision-making.
Reinforcement learning enables chatbots to adapt to changing user preferences and optimize their performance in dynamic environments. It is particularly useful in scenarios where there may not be a single correct answer, such as when providing personalized recommendations or making complex decisions.
Natural Language Processing (NLP) in Chatbot Development
Natural Language Processing (NLP) is the cornerstone of chatbot development, enabling them to understand and generate human language effectively. NLP encompasses a range of techniques and components that make chatbots fluent in human conversation. Here are key NLP components and their roles in chatbot development.
1. Tokenization
Tokenization is the process of breaking down text into individual tokens, which are typically words or subwords. Chatbots use tokenization to understand the structure of user input, enabling them to analyze and respond to text effectively.
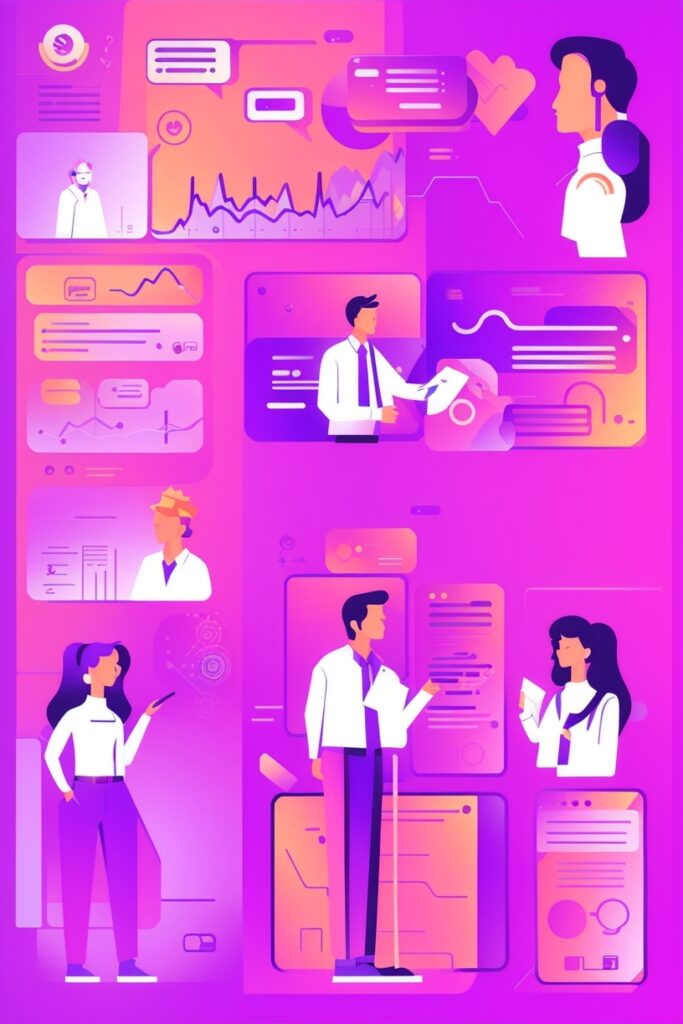
2. Named Entity Recognition (NER)
NER is a technique used to identify and categorize specific entities, such as names of people, places, organizations, dates, and more, within text. Chatbots use NER to extract relevant information from user queries, enabling them to provide context-aware responses.
3. Sentiment Analysis
Sentiment analysis, also known as opinion mining, involves determining the sentiment or emotional tone expressed in text (e.g., positive, negative, or neutral). Chatbots utilize sentiment analysis to gauge user emotions and tailor their responses accordingly.
4. Part-of-Speech Tagging (POS)
POS tagging assigns grammatical labels to words in a sentence, such as nouns, verbs, adjectives, and adverbs. Chatbots use POS tagging to analyze sentence structure and extract meaning from text.
5. Language Models
Language models, such as GPT-3, are pre-trained neural networks that excel at understanding and generating human-like text. Chatbots leverage these models to generate coherent and contextually relevant responses, enhancing the quality of their conversations.
In summary, NLP techniques empower chatbots to process and understand human language, making them effective communication tools in various domains. These techniques enable chatbots to decipher user intent, extract valuable information, and generate responses that resonate with users.
Machine learning, along with NLP, forms the backbone of modern chatbots, enabling them to adapt, learn, and provide valuable assistance across a wide range of applications and industries. In the subsequent sections of this article, we will explore the transformative impact of large language models, such as GPT-3, and how they are revolutionizing the field of AI chatbots. Stay with us as we continue our journey through the AI chatbot revolution.